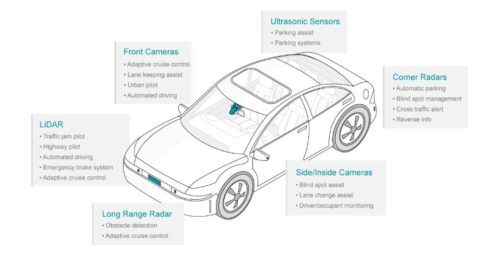
By The Numbers
Every year, more than one million people lose their lives due to crashes and road accidents. In the United States, although fatality rates declined for the first nine months of 2023, data and reports from the U.S. Department of Transportation estimated that over 30,000 people died in motor vehicle traffic crashes during that same period. This is unacceptable. The need for enhanced road safety can’t be overlooked.
The continuing loss of life on roadways has prompted new car assessment programs and governments to implement regulations and testing to better support road safety. Automotive OEMs also have an important role in designing and implementing Advanced Driver Assistance Systems (ADAS) and increasing levels of driver automation to democratize road safety.
Benefits & Potential of ADAS
The benefits of ADAS technology are clear. With multiple sensors to detect and analyze a vehicle’s surrounding environment, ADAS feeds valuable data back to the driver so they—or the vehicle’s actuation systems—can react promptly if a crash or dangerous situation occurs. According to a study conducted by the AAA Foundation for Traffic Safety and the University of North Carolina, ADAS technologies could prevent approximately 14 million injuries and 37 million crashes over the next 30 years (the 30-year period was defined as 2021 to 2050, as outlined in the study’s final technical report from the AAA Foundation for Traffic Safety).
The industry is moving steadily ahead with implementing ADAS capabilities. According to McKinsey, ADAS already represents a $55 to $70 billion revenue opportunity today. Combined with autonomous driving (AD), it could collectively represent $300 to $400 billion in revenue by 2035.
To capitalize, automotive OEMs must overcome several challenges related to sensing architecture, including whether centralized or distributed architectures offer the greater potential for their platform needs.
ADAS Tradeoffs & The Need For Sensor Fusion
Before addressing the ongoing discussion about central compute and distributed architectures, we must first understand how sensor data—both inside and outside the vehicle—works.
Both ADAS and AD leverage various sensor modalities, including computer vision, ultrasonic, radar, and LiDAR, to gather and process data about the vehicle’s exterior and interior environment. In the context of vehicle motion dynamics, this data needs to be processed to enable timely decision-making and trigger automated safety response actions such as braking, steering, or driver alerts.
However, tradeoffs exist for ADAS sensing. For example, radar can sense objects crossing or coming into a vehicle’s path across a range of weather conditions but has limited depth precision and object recognition capability. Conversely, cameras have human-like vision sensing that makes them ideal for object recognition, but they do not perform well in adverse weather and poor lighting conditions. And while LiDAR excels in range and depth precision, and is unaffected by poor lighting, it may be impeded by heavy rain or fog.
Given these tradeoffs, it is critical to bring together and process inputs from multiple types of sensors, especially for vehicles with higher levels of automation. The question then becomes which type of sensing architecture provides the best and most efficient support for all the different sensors.
Evolution of Sensing Architectures
The sensing architecture of vehicles has evolved significantly over the last decade. One trend in electrical and electronic (E/E) architecture is the consolidation of hardware into fewer electronic control units (ECUs). McKinsey explored how the industry might move towards a more centralized approach to facilitate the development of highly autonomous driving and to reduce complexity in the long run.
A few current EV models from EV-only OEMs are driven by software updates and rely on a centralized compute architecture, which offers benefits for software management and fusion-based perception. However, the high bandwidth and power consumption required for centralized processing may not make a centralized compute architecture suitable for all use cases. This is particularly true for OEMs that have legacy systems in place or more vehicle platform classes to support, such as small city cars to luxury saloons.
Centralized Compute vs. Distributed E/E Architectures
In a central compute E/E architecture, raw multi-sensor data is sent straight to a centralized system-on-chip (SoC) processor, with little to no processing occurring at the edge. By leveraging AI-enabled perception algorithms, the central compute SoC can process the sensor data and control the vehicle’s motion-related actuations. However, the architecture presents several challenges, including higher costs and limited platform scalability. Centralized architectures need powerful processing capabilities, high-speed data transfer, and significant thermal management due to the amount of power consumed in the central compute SoC.
A distributed architecture can help overcome the limitations of the central compute approach. It distributes vehicle sensing, control, actuation, and processing functions across edge, zone, and vehicle compute ECUs.
Distributed intelligence enables a more scalable architecture across vehicle classes, potentially reducing system power and costs. Reduced processing power is achieved because various sensor data can be processed closer to the sensors at the vehicle edge. This creates real-time feedback and perception, and the resulting pre-processed data is aggregated into zones for further downstream processing and perception. This approach reduces the transported raw sensor data volume and alleviates some of the interfacing, cabling (cost and weight), and thermal challenges often encountered in a central compute architecture.
In addition to platform scalability, distributed intelligence addresses interoperability head-on, helping better answer OEMs’ wider platform needs. It also enables the optionality of ECUs and ADAS sensors to meet automaker vehicle model price points.
Moving The Industry Forward
The limitations of a central compute-based architecture mean that it isn’t a one-size-fits-all solution for all automakers.
The benefits of a distributed E/E architecture—its ability to ease system design, integration, and cost challenges—are being revisited by the automotive industry. As a result, semiconductor vendors are innovating to develop edge-based vision sensing and perception solutions that enable distributed intelligence, helping OEMs make better decisions about their vehicle platform needs.