Monitoring and evaluating automated driving systems (ADS) remotely is a reality that means personal driving data is being sent to the cloud. Once there, that data helps developers and manufacturers improve the safety performance of their ADS systems. Could your personal driving data save a life?
If you own or plan to own a vehicle with an ADS, it’s important to be aware that your car only knows so much. It only knows what humans have told it to know. It uses the data on-hand to make its best guess about how to handle what it doesn’t know. The result can be good or bad.
OTA Updates & Environmental Conditions
One of the ways your personal driving data will make a difference – in terms of greater road safety and saving lives – is by adding previously unknown road situations and environmental conditions to the ADS machine learning model at hand. Such updates benefit all vehicles with that particular ADS. So, if your car sees something its system has not accounted for in the past, that information is sent back to the developer or manufacturer of your ADS, the machine learning model is updated, and everyone who owns a car with that ADS gets the update.
Say, for example, an ADS-dedicated vehicle is taking you to your destination, and you come upon a police officer in a yellow hooded poncho standing in the middle of the road directing traffic on a rainy day – if your ADS is unfamiliar with that particular “environmental condition,” the ADS developer or manufacturer would potentially get an alert to evaluate your remotely monitored data. If it’s new information, it would be added to the ADS machine learning model in the system’s next software update. It would then become a known environmental condition that requires the vehicle to stop and wait to be waved forward by the officer. Everyone else who has a vehicle with that ADS system would get the same update over the air (OTA). All of those vehicles (yours included) would now know what to do when they see something similar.
But how is this data collected? And what are manufacturers looking for in the data they collect?
UL 4600 Safety Performance Indicators
Let’s start at the beginning. In the olden days, 2019, and before, when vehicles with autonomous driving systems were deployed, there was no formal standard for updating an ADS when things went awry. Thus, in 2018, when an Uber in self-driving mode hit and killed a pedestrian pushing a bike, there was no formal safety standard to direct how that circumstance would be handled to prevent such an incident in the future.
Autonomous-system safety standard ANSI/UL 4600 addressed this issue head-on. It was issued by Underwriters Laboratories Standards & Engagement (ULSE) and introduced in 2020. It was updated in 2022 and then again in 2023. Commonly known as UL 4600, it is the first comprehensive standard for public road autonomous vehicle safety to cover urban and highway use cases. It applies to any system that allows human drivers to take their eyes off the road, including autonomous cars, automated and connected vehicles, and related products. It also provides a starting point for ensuring the safety of other autonomous ground, air, and underwater drones and vehicles.
A central concept in UL 4600 is continuous monitoring of the safety aspects of the ADS using metrics known as Safety Performance Indicators (SPIs).
What Are Safety Performance Indicators?
An SPI is a metric plus a threshold that makes it possible to assess whether a system is safe or not. As an example, if one autonomous car in a billion runs over its owner’s foot when summoned from a parking spot, that is unfortunate but perhaps socially acceptable. But if that happens once per a hundred summons, clearly, that is a problem, and something needs to be improved. Somewhere in the middle of those two extremes is a threshold set by the manufacturer for how often that and other hazardous events are permitted to happen. No car will ever be perfectly safe, but they need to be safe enough to be acceptable.
If too many unsafe actions are detected, something needs to be improved. In this case, perhaps the developers need to add an ultrasonic foot detector to ensure the car stops before it reaches the owner’s foot. (Sadly, this is a relevant example. As recently reported by Jonas Rest in Germany’s Manager Magazine, Tesla Model 3s continue rolling for up to eight seconds after being summoned to a stop. So far, no feet have been run over.)
What Are Safety Cases?
To achieve the standard – i.e., to be UL 4600 conformant – developers and manufacturers must establish what’s called a “safety case” for their ADS system. A safety case, per UL 4600, is a structured argument supported by evidence that provides a compelling, comprehensible, and valid case that a system is safe for a given application in a given environment. Developers and manufacturers must gather evidence for a safety case for their ADS and validate it before it’s deployed. They must also collect data and update the safety case after it’s deployed.
While UL 4600 defines the safety standard for autonomous systems, it does not tell developers and manufacturers how to achieve it – i.e., what process to follow, explained Dr. Philip Koopman, a professor of electrical and computer engineering at Carnegie Mellon University and author of the original UL 4600 standard proposal.
That’s where organizations like the Automated Vehicle Safety Consortium (AVSC) come in. AVSC is an industry program of the Society of Automotive Engineers Industry Technologies Consortia (SAE ITC). The core members are Aurora, Cruise, GM, Honda, Lyft, Motional, Uber, Volkswagen, and Waymo. One of the areas AVSC focuses on is proposing best practices that can be followed by companies as-is and serve as the starting points for more formalized industry standards.
AVSC00011202307 Best Practice
Recently, AVSC proposed a best practice called AVSC00011202307 for the continuous monitoring and improvement of autonomous systems after deployment. “Changes regularly occur to the operational environment after deployment,” said AVSC director Darcyne Foldenauer. “So, it makes it necessary to identify and address any new risks that arise.”
“Maintaining AI is just as important as creating AI,” wrote Dr. Missy Cummings in her recent article, What Self-Driving Cars Tell Us About AI Risks, for IEEE Spectrum. Dr. Cummings is a professor in the Department of Electrical and Computer Engineering and the Department of Computer Science at the Duke Institute for Brain Sciences (DIBS) at Duke University. She has 20 years of experience designing and testing unmanned systems and recently worked with the U.S. National Highway Traffic Safety Administration (NHTSA) as a senior safety advisor. “Because neural networks can only be effective if they are trained on significant amounts of relevant data, the quality of the data is paramount,” she wrote. “But such training is not a one-and-done scenario: Models cannot be trained and then sent off to perform well forever after. In dynamic settings like driving, models must be constantly updated to reflect new types of cars, bikes, and scooters, construction zones, traffic patterns, and so on.”
The AVSC00011202307 authors concur and offer the best practice as a method for approaching the problem. ADS developers and manufacturers should continuously monitor fleet performance and enact prompt improvements when issues arise, they wrote in their press release. Only then will they be able to scale their solutions, allowing their technology to realize its full potential.
“Insights gained about the operating environment can support manufacturer or fleet operator’s continuous improvement of ADS-dedicated vehicles safety performance after deployment,” Foldenauer explained. “They can use the data collected from vehicles in active deployments to proactively confirm initial risk assumptions and feed other safety management processes.”
I asked Dr. Koopman to weigh in on AVSC00011202307. He explained that it offers developers and manufacturers a process that can fulfill part of the requirements for the UL 4600 to implement SPIs. “It emphasizes environmental changes, which is their intention,” he said. “I’m glad to see this group make a concrete proposal in that area.”
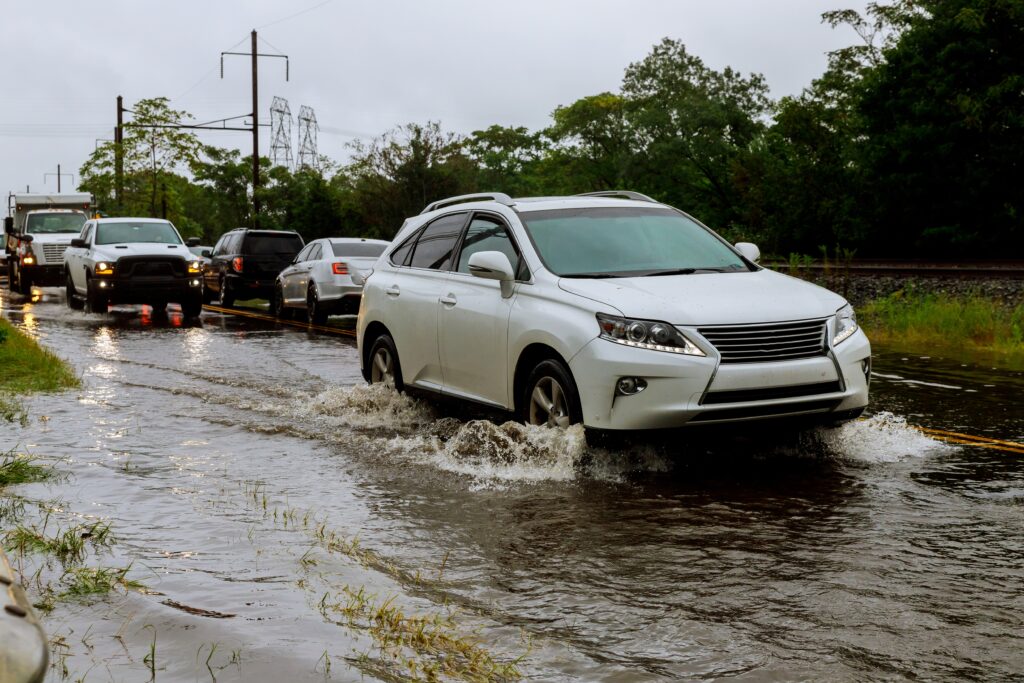
Adjusting For Unknown Environmental Changes
What are environmental changes? There are two kinds: known and unknown. The difference is whether or not the ADS development team knew about and planned for the potential change. For example, it’s known that the weather will change. Developers do their best to set their ADS system up for success by ensuring it can perform on any road in any weather condition, including rain, fog, and snow. On the other hand, it might be unknown that a bridge could collapse. While an ADS system might be proficient at crossing bridges, even in severe weather, it’s possible no plan has been made for if a section collapses due to flooding.
Known environmental changes are things development teams are aware of that are likely to change. These include updates to speed limits or road markings. They also include changes in road conditions or traffic patterns due to weather or construction. Meanwhile, unknown environmental changes include unusual debris in the road or detours due to accidents. Importantly, they include edge cases or anomalies, like a woman pushing a bicycle, as in the 2018 self-driving Uber incident.
To take the explanation a touch further and to showcase how the AVSC proposal is an important step forward, there might be things in the known category that can actually pose a hazard but have yet to be addressed in the ADS because they haven’t caused a problem, yet, explained Dr. Koopman. Let’s say, for example, developers of an ADS establish a known assumption that trees exist along roadways but not in roads. During the 10-year design, development, and deployment lifespan of their ADS system, a tree has never been a problem. Their ADS system tells its vehicles to drive on by. But what if the ADS system suddenly encounters a tree in the middle of a road? What if a tree topples into the road or drops a large limb just as an ADS-dedicated vehicle approaches? Such circumstances could qualify as unknown environmental changes that must be addressed.
AVSC, with its new best practice, proposes the conceptual structure or framework ADS developers and manufacturers can rely on to continuously monitor and improve safety performance in the presence of these known and unknown environmental changes. Doing so is a key aspect of the broader safety assurance framework for building a safety case for ADS-dedicated vehicles, Foldenauer explained. “AVSC00011202307 provides clear examples of data sources, collection methods, and analysis of data to identify areas of improvement,” she continued. “Each of our consortium members signs up to implement AVSC best practices in some manner. So, either they’re using them directly, using something similar, or working toward implementing.”
“It also helps with conformance to UL 4600’s requirement to provide continuous monitoring and feedback for the whole safety case by addressing a key component of that feedback,” Dr. Koopman added.
How Do Autonomous Driving Systems Use Data?
Personal driver data is collected and used all the time. If you’re in a newer vehicle and come up too fast and too close to the car in front of you, your automatic emergency braking (AEB) might put on the brakes to avoid the collision. If you are in a traditional car, that data – taken from sensors, including cameras, radars, and lidars – stays in your vehicle’s computer (i.e., at the edge). No updates happen to your ADAS system, and the next time you make that same maneuver, you’ll get the same result.
If you are in a connected, ADS-dedicated vehicle, that driver data doesn’t just stay within your vehicle’s computer. Rather, it’s communicated to the developer or manufacturer, who then analyzes it. The way data is communicated differs by manufacturer and operating design domain (ODD), Foldenauer explained. The AVSC best practice calls for continuous monitoring and offers flexibility on which data is collected, she noted, adding, “Continuous is not intended to imply constant. It refers to periodic monitoring established by each organization as appropriate for the scope of their deployment.”
I was curious to know if all drivers within an ADS developer or manufacturer’s fleet are included in the data collection pool. “That is up to the ADS developer or fleet manufacturer to determine,” Foldenauer said.
What Are They Looking For?
Developers and manufacturers are scanning your driving data for known and unknown environmental changes. They are comparing new data – i.e., known and unknown incidents captured by your vehicle’s sensors (and the sensors of other vehicles) – to the assumptions they made during the design of their system. On the one hand, they are “proactively confirming some of their initial risk assumptions and then feeding those results into other safety management processes,” Foldenauer said. On the other, they’re looking for flaws or reasons to update their ADS machine learning model to improve the safety of their fleet.
Why Are They Looking For That?
Back to the example of coming up too fast and too close to the car in front of you.
“In a continuously monitored fleet of ADS-dedicated vehicles, data showing that such near misses are happening too often – across all the cars of that type – would prompt an engineering change to avoid even having a near miss in the future,” Dr. Koopman said. “Safety is ultimately about converting unknowns into knowns so that hazards can be mitigated.”
Continuously monitoring and improving behavioral competency is crucial in developing a comprehensive safety assurance framework for autonomous driving systems, AVSC wrote in their press release. “This best practice helps to provide an approach that enables continuous improvement of safety performance, and we do touch on the identification of unknowns and regularly evaluating the validity of assumptions made about ADS-dedicated vehicles’ operating environment,” Foldenauer said.
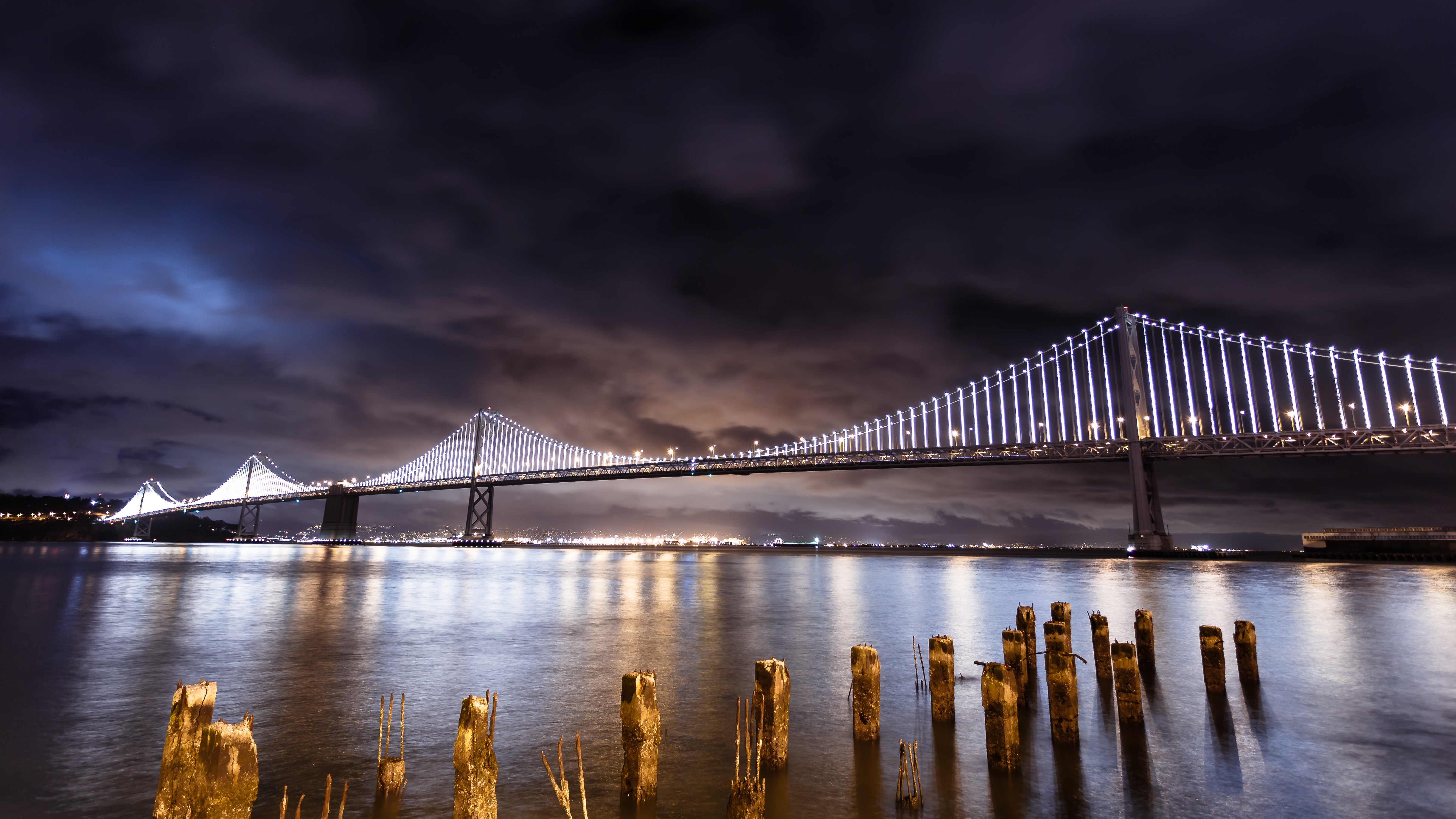
Fostering Public Trust & Acceptance
AVSC best practices are technology neutral – meaning they describe the “what” and leave the “how” for each organization to determine – and available at no charge to members and anyone else wishing to review or use them, Foldenauer explained. “We want to promote public trust in autonomous vehicles, so that’s a big part of why they’re provided for free,” she said.
“Our hope is that we will accelerate formal global standards, so the best compliment we could have is when somebody takes one of our best practices and starts to develop it into a standard,” Foldenauer continued. For example, the On-Road Automated Driving Committee (ORAD) — an SAE International committee — is planning to create a standard based on an AVSC best practice for adapting a safety management system for ADS, she explained. “ADS developers and manufacturers should strive to continuously improve upon safety performance over time and strengthen their confidence in their design assumptions,” said said.
Well before connected autonomous driver systems were a twinkle in developers’ eyes, the upper deck of the San Francisco–Oakland Bay Bridge collapsed due to an earthquake during the 1989 World Series. Human drivers on the bridge careened into the abyss. 42 people died. This event was so shocking that it was played repeatedly on national television. I want to think ADS developers have planned for this type of event and that we don’t have to wait for it to happen again for it to be included as a known environmental condition in their machine-learning models. One of the goals of UL 4600 is to capture these past pre-ADS experiences so that developers can address them. Let’s hope they are doing that.