Algolux announced the next generation of its Eos Embedded Perception Software during the recent 2020 Embedded Vision Summit. The expanded Eos portfolio, according to Algolux, leverages new AI deep learning frameworks to improve system scalability and reduce development costs for teams working on the latest future mobility and smart city applications.
“For many of these applications, there are safety-critical aspects to consider,” explained Dave Tokic, Vice President of Marketing & Strategic Partnerships for Algolux. “Whether it’s for ADAS or autonomous vehicles, or whether it’s for a security camera or a fleet operator, when they apply computer vision technologies, they need to be able to achieve high levels of accuracy.”
Key Challenges & Considerations
This new generation of Eos Embedded Perception Software is defined by a reoptimized end-to-end architecture, combined with a novel AI deep learning framework. Algolux says breakthroughs in deep learning, computational imaging, and computer vision allow the expanded Eos portfolio to improve accuracy up to three times more than expected, even in difficult operating conditions.
“People drive in the rain, they drive at night, and these systems will be used during snow,” Tokic said. “We are uniquely focused on addressing these particular challenges.”
During a recent media event hosted by Algolux, Tokic pointed to a study from AAA, published in August, that examined the effectiveness of current ADAS technology. AAA selected a handful of popular vehicles and subjected them to 4,000 miles of real-world driving. On average, the ADAS applications on the tested vehicles experienced a performance-related issue every eight miles. AAA conducted a similar study in 2019 that showed how current emergency braking and pedestrian detection systems are ineffective at night.
“We are challenged with making sure these systems are safe, and there is a critical opportunity here to save lives,” Tokic said. “These studies provide a representative benchmark of where we are as an industry.”
Addressing The Limitations
The expanded Eos portfolio is meant to address the performance limitations of current vision architectures and supervised learning methods, including biased datasets that do not account for challenging scenarios like low light and bad weather. “It’s easier to capture a dataset in good lighting and imaging conditions, so you have a bias with these datasets towards good imaging conditions,” Tokic added.
The reoptimized end-to-end architecture and novel AI deep learning framework of the expanded Eos portfolio is designed to overcome such limitations. By doing so, Algolux says customers will conserve valuable resources when it comes to training data capture, curation, and annotation costs per project.
During the recent media event, Tokic explained how this next generation of Eos Embedded Perception Software enables end-to-end learning of computer vision systems. This effectively turns them into “computationally evolving” vision systems by applying computational co-design of imaging and perception, an industry first. According to Algolux, the approach allows customers to adapt their existing datasets to new system requirements, enabling reuse while also reducing effort and cost compared to existing training methodologies.
“One of the challenges here is the tendency to train the next generation of computer vision models with the previous generation of the datasets, and whatever open-source datasets that can be aggregated relevant to the scenario,” Tokic said. “And all of that is typically captured on a camera different than the one being deployed, so the noise profile and lens characteristics are different than when that dataset was originally captured.”
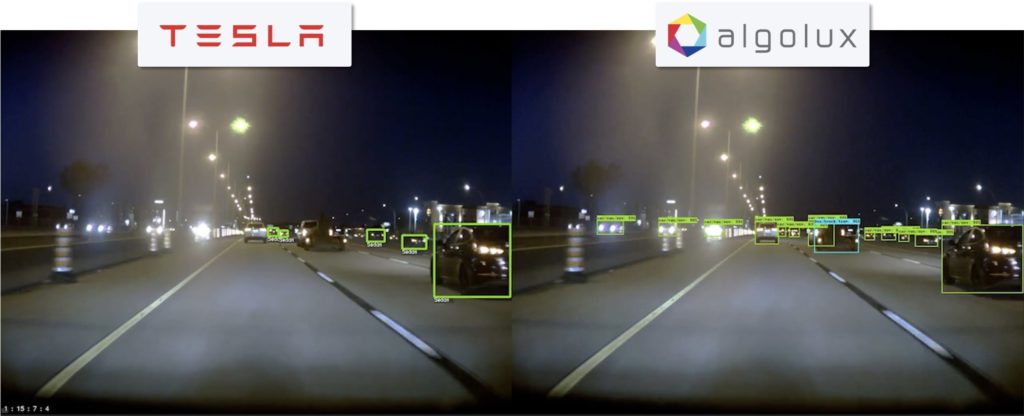
Synthesizing Broader Datasets
With the expanded Eos portfolio, the sensing and processing pipeline is included in the domain adaptation, which addresses typical edge cases in the camera design ahead of the downstream computer vision network. Unlike existing sequential perception stacks that tackle edge cases purely by relying on supervised learning using large biased datasets, the AI framework “learns” the camera jointly with the perception stack to address any limitations.
“This training framework data takes the existing jpg. data and transforms or adapts it back into a raw form, specifically based on the characteristics that we capture through a calibration process of the new lens and sensor with the customer. It’s as if their old training dataset was captured by the new camera,” Tokic explained. “We can simulate or synthesize a broader dataset based on the characterization of that lens and sensor to cover as if the dataset was captured in low lighting or high noise, all the way up to good imaging conditions. This allows us to explore the entire operating range of that lens and sensor combination.”
Eos Availability
The expanded Eos portfolio from Algolux is available now. The Eos set of perception components can address individual NCAP requirements, Level 2 and above ADAS features, higher levels of autonomy, and video security and fleet management.