Algolux has unveiled its new Atlas Camera Optimization Suite, a comprehensive set of machine-learning tools and workflows that automatically optimize camera architectures for computer vision. According to Algolux, object detection or instance segmentation results improve by up to 30 mean Average Precision (mAP) points with the Atlas Camera Optimization Suite. Similarly, Algolux says the new suite produces computer vision results in days versus manual camera tuning approaches, which can take weeks or months.
“The Atlas Camera Optimization suite was first created to address a critical market need,” explained Dave Tokic, Vice President of Marketing & Strategic Partnerships for Algolux. “Despite cameras being the sensor of choice for safety-critical applications, such as ADAS and AVs, system developers still rely on expert imaging teams to manually tune camera architectures to achieve good image quality.”
Tokic notes how this traditional process takes an extended amount of time, requires difficult-to-find expertise, and is dependant on visual subjectivity. “As such, this process does not ensure that the camera provides the optimal output for either display purposes or for computer vision algorithms and is inherently not scalable,” he said.
Camera Tuning vs. Optimization
Tuning is a manual process that requires deep domain expertise and experimentation to find a “good” visual result, but there is no way of knowing whether the ISP parameter set is optimal for computer vision. Also, what works for one computer vision model may not apply to a different model.
On the other hand, Optimization is a dynamic and automated process and is much faster and scalable. Via Atlas, it is also applicable to any camera configuration specific to any target vision model or ensemble of models.
~ Dave Tokic, Vice President of Marketing & Strategic Partnerships for Algolux, on the difference between camera tuning and optimization.

Atlas Camera Optimization Suite: How it Works & What it Does
Digital cameras today consist of a lens, sensor, and an image signal processor (ISP) with a chain of processing blocks like denoising, sharpening, and color correction. These serve to convert the raw sensor data into an appealing image. Each ISP block has parameters that adjust the output of that block, adding up to many hundreds of parameters, Tokic explained.
Imaging experts then capture lab and field test images and manually modify those parameters over months to achieve the right image for the application. “While this painful ‘golden eye’ approach can produce a good visual image, it is not scalable and cannot determine if the camera output is optimal for any specific computer vision task,” Tokic continued. “And there is no way for the team to know what is best for the vision algorithm.”
The Atlas Camera Optimization Suite is used during the vision system development process to identify the camera’s optimal ISP parameter set for that lens/sensor/ISP combination and the computer vision algorithm. Primarily, the Atlas Camera Optimization Suite searches for the right ISP parameter set that maximizes the computer vision metrics in question.
“This is done quickly and automatically by iteratively injecting a small dataset of annotated raw images into the ISP and using that as an input to the computer vision task(s) being optimized,” Tokic added. “The development team lets Atlas explore this parameter space until it finds the optimal set.”
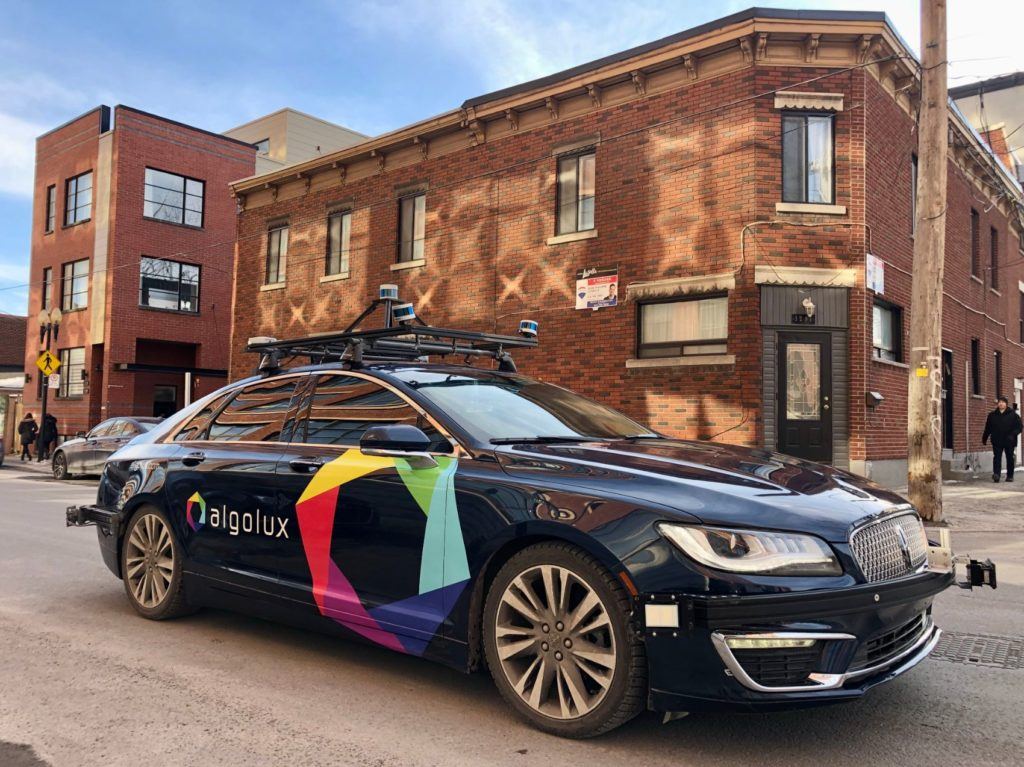
Inspired by Research
Algolux developed the Atlas Camera Optimization Suite after researching ways to improve things like camera optimization and computer vision. The findings were given as an oral presentation during the 2020 Conference on Computer Vision and Pattern Recognition.
“The researchers improved end-to-end losses compared to manual adjustment and existing approximation-based approaches,” Tokic said of the presentation. “This was done for multiple camera configurations and computer vision models such as object detection, instance segmentation, and panoptic segmentation.”
Specifically, for automotive 2D object detection, the new method outperformed manual expert tuning by 30 percent mAP and recent methods using ISP approximations by 18 percent mAP.
A total of six research papers from Algolux were ultimately accepted at the 2020 Conference on Computer Vision and Pattern Recognition.”For a company of our size, this is a massive achievement and it really highlights the quality of the work from our team,” Tokic said.
Earlier this year, Algolux shared other critical insights from their team on ADAS technology with AutoVision News, including the importance of training data and how camera images are well-suited for the human eye, but not necessarily so for machine vision algorithms.